3 Questions To…. Marion Bergeret, a Paris & New York bar qualified tech enthusiast, former lawyer, now VP Legal & General Counsel at Snips, a platform that allows any company with a device to create a private-by-design voice assistant that runs on the edge.
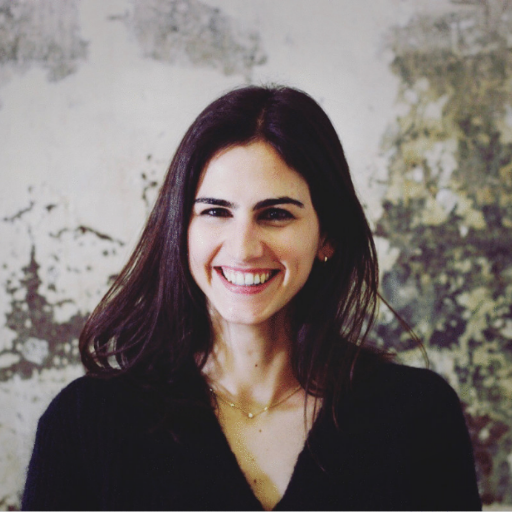
1.
Have you heard? This is the silent sound of your voice assistant listening to your intimate family and business conversations. As was recently exposed in the news, Amazon Alexa, Apple Siri or Google Home might record conversations without users being aware of it, and allegedly keep voice recordings indefinitely, or sell them to contractors. As a specialist of Voice AI, what would you say are the main challenges facing voice recognition technology today?
Most of the latest “scandals” in the voice recognition space have come as a surprise to most people working in the space, including at Snips. The level of public outrage at the news of Amazon, Google or Apple “listening” to voice recordings in order to label the voice data they collect only serves to highlight the layman’s general lack of awareness and education about how the services and devices they use actually work.
Most people familiar with voice recognition understand that voice recordings cannot be used to train algorithms unless they are “labeled”. Depending on what they are used for, to “label” voice recordings means to transcribe them into text, or to identify their meaning and structure in a systematic way using meaningful labels. In the current state of voice recognition technology, this usually involves a human listening to voice recordings and writing them out or adding the right labels to the voice segments in the context of what is called “supervised learning”.
Currently, the most widespread solution to labeling massive amounts of data is to have it done by humans, and usually this is outsourced to companies who specialise in these types of tasks for cost-efficiency purposes. And so it should come as no surprise that all the aforementioned voice assistant providers do this with the data they collect - otherwise those data would be worthless.
Obviously there should be safeguards in place (contractual, technical, organisational) to make sure that outsourcing these tasks to humans does not create privacy leaks, but it should be clear by now that humans labeling data is both common practice and not prohibited by any law.
Educating the general public to the level of general understanding of technology they need to be able to make decisions regarding their personal data is the first priority.
Educating the general public to the level of general understanding of technology they need to be able to make decisions regarding their personal data is the first priority. Until we achieve that ground level of understanding, all the transparency, information, and consent requirements embodied in the GDPR and other regulatory instruments will be largely meaningless.
2.
One of the issues at stake seems to be the storage of voice data in the cloud. Indeed, most providers offer centralized cloud-based platforms, it is thus difficult for users to fully understand and control what will happen to their data. On the other hand, you claim that removing the risks of spying or leaking is possible, if we keep data on device thanks to embedded voice recognition solutions. Could you explain to us how such solution works, and how an AI system can operate effectively without storing and learning from this data?
Most voice recognition providers claim that centralising end user data is required in order to maximise the performance of their cloud-based assistants. In other words, they claim that their technology would not work if they did not collect massive amounts of voice recordings centrally in the cloud.
At Snips, our team of machine learning experts have proven this to be untrue. In the realm of voice recognition, performance is in fact only minimally improved with the collection of more data past a certain point, and systematic recording with the goal of improving models is not necessarily justified. Snips has actually achieved performance close to human level on certain use cases, with results higher than or on par with Google’s cloud-based services, thus showing that massive volumes of voice recordings are not necessary to achieve high performance.
To make sure it is able to safeguard end user privacy whilst also ensuring performance on par or better than the main cloud-based providers, Snips uses crowdsourced training data, as opposed to end user voice queries, to train its algorithms. These are data collected from a series of providers and crowdworkers for a price. In addition, Snips uses the crowdsourced data to pre-train its voice interfaces on the use case most relevant to the device at use (rather than using a general model like most other providers), and further optimizes all the inference processes. This allows a stable, high-performance voice interface to then be embarked on a device irrespective of whether the device is connected to the internet. The voice recognition process then takes place entirely on the device without requiring powerful servers. The voice interface’s performance is already at state-of-the-art level at the time when it is added to the device, and it does not need to “improve” every day on the basis of end user data.
3.
You are convinced users do not have to trade privacy for innovation. In your view, what should be done to make sure this is true? What are the next privacy battles: implementation of technical standards such as advanced encryption, new regulation, ethics… ?
Transparency in terms of the actual technical purposes for which data are collected should be the first priority. It is not ok for individuals who use voice interfaces on a daily basis not to understand how these work, and how their data are used.
The first determining factor in increasing transparency will be enforcement of the principle by which companies must clearly state the purpose for which they collect data, so that end users are able to make an informed decision.
That will not solve the problem entirely, as even with regulation on transparency like the GDPR, enforcement will necessarily be subjective and uncertain (what counts as “sufficiently clear” information? What design choices can or cannot be used to “nudge” user behavior in the direction most favorable to the provider? etc.).
The next battle will be an ethical one. The most important question is not whether a given provider is compliant with the GDPR or not - and technically, cloud-based voice recognition can be completely compliant with the GDPR if it respects its transparency and other requirements.
The most important question is whether we think, as a society, that we should be encouraging the massive collection of personal data that is taking place through voice assistants, even though alternatives with zero privacy impact actually exist, with the same technical performance level. That is not a compliance question but an ethical one, and the GDPR is not very helpful in addressing it.
Access to the best-performing, most secure, most trustworthy voice recognition technology should come at no privacy cost to end users, especially when used in the context of their own home or in a professional context.
Access to the best-performing, most secure, most trustworthy voice recognition technology should come at no privacy cost to end users, especially when used in the context of their own home or in a professional context. Solutions are being developed through a combination of research into privacy-preserving machine learning techniques and educating consumers, who will in turn demand that their privacy not be impacted any further than is actually necessary to provide the service they requested.
Snips has shown that voice recognition can be done entirely on-device, from wake word to automatic speech recognition, and through to natural language understanding, with no impact on either performance or end user experience.
Other developments are also being experimented with at the moment with privacy in mind. Homomorphic encryption allows a server to run operations over encrypted data without decrypting them, which means there can be no privacy leakage. Once this type of technology reaches production-level, cloud-based voice recognition will become possible with less impact on privacy.
TO GO FURTHER:
What challenges or considerations arise from relying on human involvement in labeling voice recordings for algorithm training?